about
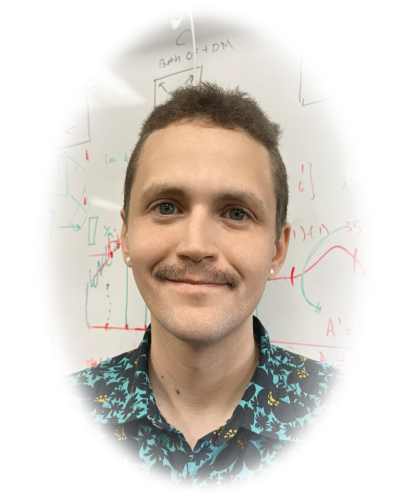
I began my career with a focus on behavioral and cellular neuroscience, specifically trying to understand what governs the rich dynamics of synapses in the brain. With a wide arsenal of experimental techniques, I then transitioned to studying how the visual system performs computations to extract meaningful information from the high-dimensional stimuli encountered by an organism in naturalistic environments. Towards answering this question, I became deeply interested in the interpretibility of modern machine-learning models, and the reciperocal relationship they offer neuroscientists:
1. Better understanding the mechanisms of the brain may yield more performant and efficient machine-learning systems
2. Powerful modeling techniques pioneered by the machine-learning community can aid in the understanding of new computational mechanisms at work in the brain.
My current research interests lie at the intersection of computational-neuroscience and machine-learning. I am particularly interested in:
- understanding the role that dynamics play in compressing and sharing information in biological and artificial neural networks
- using neuroscience frameworks to better understand the intermediate computations of large "black-box" neural networks
- using modern experimental advances to identifying principles of neural computation that have not-yet been adopted by the machine-learning community
- generating strategies (both de novo and biologically-inspired) for generating more light-weight neural networks that can do more with less